June 12, 2023
Author: Manish Verma
Editor: Dr. Jitendra Kumar Sinha
Learning is a complex process that involves changes in the brain’s synapses, which are tiny structures responsible for communication between neurons. These synapses, located in the central nervous system, are challenging to study in vivo due to their small size and high density. However, a recent breakthrough in the field has allowed researchers to overcome these limitations and directly relate receptor dynamics to animal behavior.
In a groundbreaking study, a team of scientists developed a novel approach that combines computational and biological methods to investigate synaptic plasticity. Their innovative technique not only enhances our understanding of the underlying mechanisms of learning but also offers new avenues for studying the brain.
The key to this breakthrough lies in a deep-learning image-restoration algorithm, termed as Cross-trained CARE (XTC) algorithm, which the researchers trained meticulously. This algorithm harnesses the advantages of both ex vivo super-resolution imaging and in vivo imaging modalities, to restore low-resolution in vivo 2p data obtained from a genetically modified mouse line known as SEP-GluA2. By doing so, it overcomes the limitations specific to each optical system, enabling a more comprehensive understanding of synapses. This approach allowed for the visualization of individual excitatory synapse strength over an extended period.
The first step in this approach involved training the deep-learning algorithm using ex vivo data. This ex vivo data provided high-resolution images of synapses, which are difficult to obtain directly in living organisms. By leveraging the advantages of ex vivo super-resolution imaging, the algorithm learned to restore images and resolve synapses with remarkable precision.
With this trained algorithm in hand, the researchers shifted their focus to in vivo imaging. Their focus centered on transgenic mice that expressed fluorescently labeled glutamate receptors, enabling the visualization of these vital synaptic components. When the image-restoration algorithm was applied to the in vivo images, it yielded a stunning result – the super-resolution of synapses in living animals. With the ability to visualize and track behavior-associated synaptic plasticity at a high spatial resolution, this innovative method opens up new possibilities for understanding the relationship between learning and changes in glutamate receptors. Researchers can now study the intricate details of synaptic modifications and their correlation with animal behavior more precisely than ever before.
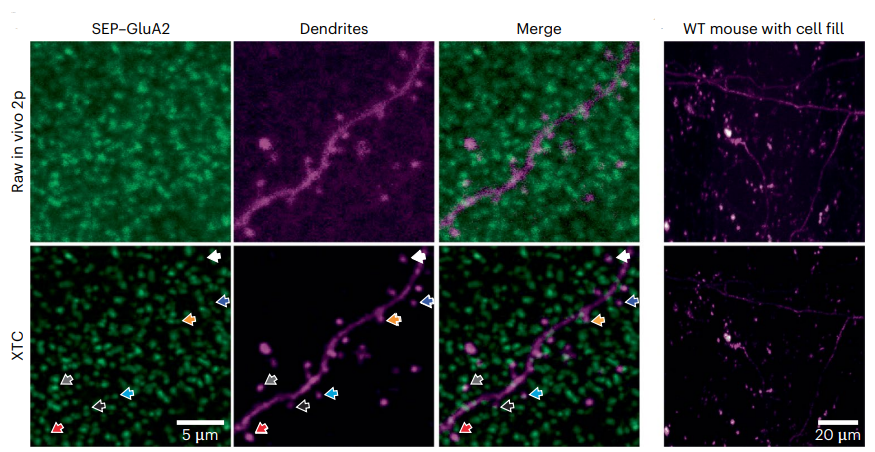
[Source – directly reproduced from Xu et al., 2023]
Compared to existing state-of-the-art image-denoising algorithms, XTC demonstrated superior performance, enabling reliable longitudinal tracking of synapses in regions with high synapse density (see figure above). By combining the strengths of various imaging modalities, deep-learning techniques, and transgenic labeling, this platform offers a versatile approach for studying objects that are close to the diffraction limit in vivo. Furthermore, it provides researchers with a valuable tool to investigate the involvement of synaptic plasticity in learning and memory at a high resolution.
This study highlights the power of combining computational techniques with biological research. By leveraging the capabilities of image enhancement algorithms and integrating them with in vivo imaging, researchers can obtain valuable insights into biological processes that were previously unattainable. This interdisciplinary approach has the potential to revolutionize not only the field of neuroscience but also other areas of biological research.
In summary, the development of a deep-learning image-restoration algorithm has ushered in a new era of studying synaptic plasticity and learning. By combining computational and biological methods, researchers have overcome the limitations of in vivo imaging and achieved super-resolution of synapses in living organisms. This breakthrough paves the way for a deeper understanding of the intricate mechanisms underlying learning processes and offers new opportunities for exploring the mysteries of the human brain.
References:
- Xu, Y. K. T., Graves, A. R., Coste, G. I., Huganir, R. L., Bergles, D. E., Charles, A. S., & Sulam, J. (2023). Cross-modality supervised image restoration enables nanoscale tracking of synaptic plasticity in living mice. Nature methods, 20(6), 935–944. https://doi.org/10.1038/s41592-023-01871-6
- Miesenböck, G., De Angelis, D. A., & Rothman, J. E. (1998). Visualizing secretion and synaptic transmission with pH-sensitive green fluorescent proteins. Nature, 394(6689), 192–195. https://doi.org/10.1038/28190
- Graves, A. R., Roth, R. H., Tan, H. L., Zhu, Q., Bygrave, A. M., Lopez-Ortega, E., Hong, I., Spiegel, A. C., Johnson, R. C., Vogelstein, J. T., Tward, D. J., Miller, M. I., & Huganir, R. L. (2021). Visualizing synaptic plasticity in vivo by large-scale imaging of endogenous AMPA receptors. eLife, 10, e66809. https://doi.org/10.7554/eLife.66809
Recent Articles
.